Table of Contents
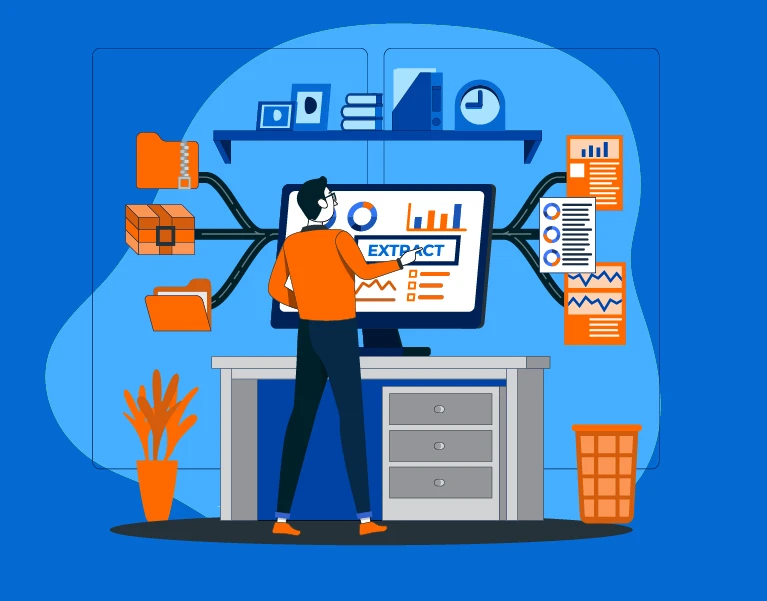
This fuzzy data match guide is created for business and tech teams that work directly with customer data and are often caught in the complexities of names, dates, phone numbers, email addresses, and location data. The guide will help answer some questions on data matching and enable teams to identify new prospects, resolve duplicate identities, and create clean customer lists using fuzzy match techniques.
Ready.
Let’s roll.
Table of Contents
What Are The Challenges With Customer Data
To understand the need and importance of fuzzy match processes, we must first address the challenges with customer data – specifically customer contact data such as names, phone numbers, email addresses, and location data that comes packed with challenges like duplicate entries, missing values, questionable attributes, false information, and multiple variations.
The image below is an example. You have different versions of a Mike Johnson whose address and phone numbers are far from accurate. He is known as Mike in the company’s CRM, but the billing team knows him as Michael, while in the vendor database, he is an M. Johnson. Which of these identities are real, complete, and accurate? Answering this mere question would require business and tech teams to spend countless hours profiling and reviewing the data across multiple spreadsheets.
Fuzzy data matching, therefore, isn’t a fancy IT technology – it is pretty much the most effective way of resolving these discrepancies and inaccuracies, going as far as unifying these disparate identities into a consolidated customer profile for business and tech teams to work on.
Other than marketing and sales, customer data is also critical when companies merge and want to combine their customer relationship management (CRM) systems. That’s when they would need to eliminate duplicates, merge records, and purge redundant entries – and attempting to do all of this on good ole Excel no longer cuts it. Teams need data match capabilities that can help them scan millions of rows of data, identifying duplicate, redundant entries within minutes – not days and months!
That’s where fuzzy data match tools and technologies come into play.
But before we talk about fuzzy match implementation techniques, let’s go through some basics.
What is Fuzzy Data Match?
Fuzzy data matching is a technique used in data preparation and analysis. It works by reviewing the similarity between two strings of text & produces a similarity score that takes into consideration factors like character overlap, edit distance, and phonetic similarity. It attempts to answer questions like:
- Is the data in Table A related to the data in Table B?
- Is Kathryn, Katherine, Catherine or Kathy the same person?
- If the data in Table A is merged with the data in Table B, will these different variations of Katherine be treated as separate records?
In simple terms, the fuzzy match is a “logic” that compares different data sets to identify duplicates and solve the given questions. Traditional deterministic methods, which rely on exact matches, often fail to identify different types of inconsistencies (as given in the image below). Fuzzy data matching techniques address these issues by evaluating the similarity between data points & assigning a similarity score.
How Does Fuzzy Match Logic Work?
Fuzzy match logic works by comparing two strings of texts and reviewing the similarity between them. For example, it will compare Kathryn vs Katherine and will analyze the level of similarity. It will then use an algorithm to assign a similarity score. In this case, if the match logic is making use of the Levenshtein algorithm, the name has a similarity score of 80-85%, which indicates that it is a duplicate!
What Are Common Fuzzy Matching Algorithms?
Fuzzy matching algorithms help businesses identify and manage inconsistencies within datasets by recognizing patterns, variations, and similarities rather than exact matches. Each algorithm has its strengths and weaknesses, making some better suited for specific scenarios and use-cases. Below, we explore several commonly used fuzzy matching algorithms in detail.
Fuzzy match theory and algorithms
1️⃣ Levenshtein Distance (Edit Distance)
This algorithm calculates the minimum number of single-character edits—insertions, deletions, or substitutions—required to transform one string into another.
👉 Formula:
Levenshtein Distance = Number of edits
Levenshtein Distance=Number of edits
⇒ Example: Transforming “kitten” to “sitting” involves three edits: ‘k’ → ‘s’, ‘e’ → ‘i’, and adding ‘g’.
Use Cases:
- Spell Checkers: Identifying and correcting typographical errors in text.
- Data Deduplication: Merging records with slight variations, such as “Jon” and “John”.
2️ Damerau-Levenshtein Distance
An extension of the Levenshtein Distance, this algorithm also accounts for transpositions (swapping of adjacent characters) as a single edit.
⇒ Example: The distance between “ca” and “ac” is 1 (one transposition).
Use Cases:
- Data Entry Correction: Rectifying common typographical errors where adjacent characters are swapped.
- DNA Sequencing: Identifying mutations that involve character transpositions.
3️⃣ Jaro-Winkler Distance
This algorithm measures the similarity between two strings by considering the number and order of matching characters, with a higher score for strings that match from the beginning.
⇒ Example: “MARTHA” and “MARHTA” have a Jaro-Winkler similarity of approximately 0.961.
Use Cases:
- Record Linkage: Matching names in databases where minor typographical errors may occur.
- Duplicate Detection: Identifying duplicate entries in customer databases.
4️⃣ Soundex
A phonetic algorithm that encodes words based on their pronunciation, converting them into a four-character code.
⇒ Example: “Smith” and “Smyth” both encode to S530.
Use Cases:
- Genealogy Research: Matching surnames with similar pronunciations but different spellings.
- Data Cleaning: Standardizing name spellings in large datasets.
5️⃣ N-Gram Similarity
This technique compares strings based on contiguous sequences of ‘n’ characters (n-grams).
⇒ Example: Comparing the bigrams (2-grams) of “night” (“ni”, “ig”, “gh”, “ht”) and “nacht” (“na”, “ac”, “ch”, “ht”) shows an overlap of one bigram (“ht”).
Use Cases:
- Plagiarism Detection: Identifying similar sequences of words in documents.
- DNA Analysis: Comparing genetic sequences by analyzing overlapping segments.
6️⃣ Cosine Similarity
Measures the cosine of the angle between two non-zero vectors of an inner product space, effectively assessing the orientation and not the magnitude.
👉 Formula: Cosine Similarity=𝐴⋅𝐵 / ∥𝐴∥ ∥𝐵∥
⇒ Example: The phrases “data science” and “science data” have a high cosine similarity because they contain the same words, just in different orders.
Use Cases:
- Information Retrieval: Determining the relevance of documents to search queries.
- Recommendation Systems: Assessing similarity between user preferences and available items.
Algorithm Comparison
Algorithm | Speed | Accuracy | Best For | Avoid When… |
Levenshtein | Medium | High | Short strings, typo correction | Long texts due to computational complexity |
Damerau-Levenshtein | Medium | High | Detecting transposition errors | Long texts due to computational complexity |
Jaro-Winkler | Fast | Medium | Name matching | Long strings with many transpositions |
Soundex | Fast | Low | Matching phonetically similar names | Non-phonetic data |
N-Gram Similarity | Medium | Medium | Text comparison | Very short strings |
Cosine Similarity | Slow | High | Document similarity | Small datasets |
Industry Applications
👉 Healthcare: Soundex can merge patient records with phonetically similar names, ensuring continuity of care.
👉Finance: Damerau-Levenshtein helps detect fraud by identifying slight variations in account details.
👉Retail: Cosine Similarity enhances product recommendation systems by assessing the similarity between product descriptions.
Understanding these algorithms and their appropriate applications is crucial for effective data management, ensuring accuracy in tasks like data cleaning, record linkage, and information retrieval.
Three Common Fuzzy Match Methods
Organizations typically implement fuzzy matching through three primary methods: Python scripting, SQL queries, and No-code software. Each method serves distinct purposes and suits different skill sets and organizational needs. Let’s explore them individually first, followed by a clear comparison table.
1️⃣ Code-Based Fuzzy Matching (Python/R)
Developers utilize programming languages like Python or R to implement fuzzy matching algorithms through various libraries.
Example in Python:
python
from fuzzywuzzy import fuzz
name1 = “Kathryn Johnson”
name2 = “Catherine Johnson”
similarity = fuzz.ratio(name1, name2) # Returns 82
print(f”Match Confidence: {similarity}%”)
Use Case:
A hospital merges patient records from multiple legacy systems:
Matches “Dr. Emily O’Neill” (System A) with “Emily Oneil” (System B) using Jaro-Winkler
Identifies over 12,000 duplicate records in 4 hours
Tools:
Python: FuzzyWuzzy, PolyFuzz, RapidFuzz
R: stringdist, RecordLinkage
2️⃣ SQL-Based Fuzzy Matching
This method employs SQL functions for basic fuzzy matching, primarily focusing on exact or phonetic approximations.
Example:
sql
— Match similar names using SOUNDEX
SELECT *
FROM customers
WHERE SOUNDEX(first_name) = SOUNDEX(‘Catherine’)
OR first_name LIKE ‘Kath%’;
Use Case:
A small e-commerce business deduplicates 500 product entries:
Links “Wireless Bluetooth Headphones” with “Bluetooth Headset” using LIKE operators
Reduces SKU count by 18%
Limitations:
Most SQL dialects lack native support for advanced algorithms like Levenshtein or Jaro-Winkler
Accuracy may be limited for complex matches
3️⃣ No-Code Fuzzy Matching
User-friendly tools, such as WinPure, automate the fuzzy matching process with prebuilt algorithms.
Example:
A marketing team imports 100,000 CRM records and uses “Fuzzy Grouping” to cluster similar entries:
“Jon [email protected]”
“Jonathan Smith (Work)”
“J. Smyth”
The team merges 2,300 duplicates in 15 minutes.
Comparison of Fuzzy Matching Methods
Criteria | Python/R | SQL | No-Code Tools |
Learning Curve | Steep (Requires coding skills) | Moderate (Basic SQL knowledge) | Minimal (Drag-and-drop interface) |
Speed | 10K records/hour | 5K records/hour | 1M+ records/hour |
Accuracy | 85-95% (Customizable algorithms) | 50-70% (Limited functions) | 90-98% (Pre-optimized models) |
Scalability | Manual scaling required | Limited to DB capacity | Auto-scales with cloud integration |
Best For | Data scientists, custom matching logic | Quick fixes on small datasets | Business teams needing fast, accurate results |
When to Use Each Method
- Python/R:
→Ideal: Research institutions matching genomic data (“BRCA1” vs “breast cancer 1”)
→Avoid: Time-sensitive marketing campaigns - SQL:
→Ideal: Small businesses deduplicating fewer than 1,000 product listings
→Avoid: Multilingual name matching (“Jorge” vs “George”) - No-Code:
→Ideal: Enterprises merging CRM systems post-acquisition
→Avoid: Highly specialized matching (e.g., chemical compound names)
Choosing the right fuzzy matching method depends on the complexity of your use-case, technical skill set availability, and speed requirements. While Python and SQL remain powerful tools for highly customized scenarios, no-code software solutions provide unmatched convenience, speed, and accessibility, making them ideal for most modern business environments.
Why You Should Consider A Fuzzy Match Tool Over Scripts & Spreadsheets
Metric | Manual Process | Automation | Expected Savings |
Time Spent (hours) | 100 | 2 | 98% time saved |
Cost (USD) | $2,500 | $300 | 80% cost saved (~$2,000) |
Effort Reduction | High (repetitive tasks) | Low (mostly review) | Significant (focus on high-value tasks) |
Organizations traditionally rely on scripts (Python, R, SQL) or spreadsheets (Excel) for fuzzy data matching. While familiar, these methods fall short in handling today’s sophisticated data challenges, creating bottlenecks in operations, data accuracy, and strategic insight generation. Here’s a strategic comparison showcasing why modern fuzzy matching tools dramatically outperform traditional methods.
Challenges Of Scripts & Spreadsheets
❌ Limited Scalability
⇒ Excel supports around 1 million rows, beyond which it becomes unstable or unusable.
⇒ Performance drastically decreases when handling thousands of records.
❌ Resource-Intensive Scripting
Developing fuzzy matching algorithms in Python or SQL requires:
⇒ Deep programming expertise.
⇒ Significant development and testing time.
⇒ Ongoing maintenance and updating of scripts.
A financial institution using custom Python scripts might spend months tuning Levenshtein distance thresholds to match transaction records, with continual issues in matching accuracy due to the complexity of financial terminologies and naming conventions.
❌ Increased Risk of Human Errors
Manual processes in Excel or SQL queries significantly increase the risk of human errors:
⇒ Typos, incorrect logic, missed edge cases.
⇒ Increased false positives/negatives, impacting critical business decisions.
❌ Limited Scalability and Flexibility
⇒ SQL databases have limited built-in fuzzy matching capabilities, often restricted to phonetic algorithms like SOUNDEX.
⇒ Implementing advanced matching algorithms (Jaro-Winkler, N-Gram) typically requires custom UDFs, which degrade database performance.
Now let’s talk about what kind of advantages dedicated fuzzy match tools bring to the table
Strategic Advantages Of Dedicated Fuzzy Match Tools
✔ Simplified User Experience
👉 No-code tools provide intuitive drag-and-drop interfaces.
👉 Users without programming knowledge (business analysts, marketers) can easily perform complex matching tasks.
✔ Superior Accuracy
Built-in advanced algorithms optimized through real-world scenarios:
👉 Jaro-Winkler for names and addresses.
👉 Levenshtein Distance for typographical errors.
👉 Double Metaphone for multilingual phonetic matching.
✅ Banks use fuzzy tools to reliably match international client names across multiple languages, significantly reducing errors in KYC compliance.
✔ Enhanced Speed And Scalability
👉 Specialized fuzzy matching software is designed for high performance, rapidly processing datasets of millions of records.
👉 Far superior speed compared to scripts or spreadsheets, which significantly reduces time-to-insights.
✅ A financial services firm processed 1 million records using a fuzzy match tool in less than an hour, compared to 5 days using traditional Python scripting.
✔ Seamless Integration
👉 Dedicated fuzzy match tools provide seamless integration with various data sources, such as CRMs, cloud databases, Excel sheets, and APIs.
👉 Automated processes can routinely identify and remove duplicates without manual intervention.
✔ Advanced Customization And Flexibility
- Fuzzy matching tools allow users to:
👉 Adjust matching sensitivity thresholds.
👉 Define custom rules for specific data types.
👉 Create personalized word dictionaries and reference tables.
✔ Built-In Data Quality & Standardization Features
- Dedicated fuzzy matching software often includes features beyond fuzzy algorithms:
👉 Data profiling for identifying inconsistencies.
👉 Data cleansing and standardization modules.
👉 Integration with official address verification databases.
✅ A healthcare provider used a fuzzy matching tool integrated with USPS to standardize patient addresses across multiple systems, reducing returned mail costs by 85%.
✔ Reduced Technical Dependency
👉 Specialized fuzzy matching tools significantly reduce dependency on highly skilled technical resources.
👉 Allows teams to focus their expertise on higher-value tasks (analytics, governance, and strategy), rather than repetitive manual data-cleaning processes.
While scripts and spreadsheets may suffice for small, simple datasets or one-off projects, specialized fuzzy matching tools offer high end benefits in terms of efficiency, accuracy, scalability, integration, and ease of use.
Strategic Comparison Matrix
Aspect | Spreadsheets & Scripts | Specialized Fuzzy Match Tools |
Implementation Burden | High (extensive programming required) | Low (user-friendly, intuitive interface) |
Data Scalability | Limited (struggles with large volumes) | Excellent (designed for massive datasets) |
Matching Accuracy | Moderate (basic or manual tuning needed) | High (advanced, optimized algorithms) |
Time-to-Insights | Slow (days or weeks) | Rapid (minutes or hours) |
Integration | Manual, isolated, or complex | Automated, seamless, extensive |
Resource Allocation | High manual intervention | Minimal manual oversight |
Adaptability | Low (manual updates needed frequently) | High (automated machine learning updates) |
Strategic Advantage | Minimal (operational burden) | Significant (strategic empowerment) |
How Is Fuzzy Match Used To Clean & Match Contact Data
Contact data is made of essentially five components – names, dates, phone numbers, email addresses, and location data.
Fuzzy matching technologies can be used to clean and deduplicate customer contact data far more effectively than Excel, Python, or SQL.
Here’s how.
Challenges With Name Matching
Names are hard. It could take you a while to figure out if Kathryn, Katherine, Kathy, Cathy, Catherine, Kath, Cath (WHEW!) are all the same person or duplicates.
In a database, names are often the first components used to compare and match identities – and are also the most complex.
Not only do you have to match first names and last names, but also suffixes and honorifics. If that’s not enough, you also have the challenge of cultural names and their variations.
Apart from individual names, you also have company or corporation names where you could also have numbers and abbreviations within the name itself – for example, IBM is an abbreviation, and, 7Eleven has a number in the name.
When these identities are duplicated or non-standardized, they become difficult to resolve, especially if the business does not have a name-matching tool.
Fuzzy name matching enables an analyst to decide whether Mrs Katherine Jones from NYC is the same individual as Ms Catherine from NY even if they have varying phone numbers or email addresses.
Additional challenges with name data that fuzzy matching can help solve:
Fuzzy name-match solutions can help with:
- Identifying duplicates in multi-cultural names
- Identifying and fixing missing names
- Identifying multiple variations of the name in your database
- Standardize names and follow a custom word dictionary (which you can build!)
- Merge all possible duplicates into groups for reviewing
A lot can go wrong with names, even if you have a well-defined system and a solid data schema, especially because it’s hardly consistent and is almost always entered manually.
You need more than just an Excel formula to handle these variations, and that’s where fuzzy data matching is the most useful.
Watch how to solve name-matching challenges with WinPure:
Phone Numbers And Numeric Matching
With SSNs, tax IDs, and sensitive identification numbers being restricted for use under data privacy laws, mobile phone numbers are the only “living” publicly available unique identifiers that can be used. But this is far from being simple and easy.
Phone numbers too have multiple formats and are often messy and varied, especially when different formats and additional information are included. Take these examples from both the US and the UK:
These examples show the variety of formats, extensions, and even notes that often find their way into phone number fields, creating a challenge for data consistency and accuracy.
Fuzzy numeric data matching can help clean up and unify these varied phone number formats by identifying similarities across different representations. For example, it can recognize that “(800) 555-1234” and “800-555-1234” refer to the same number despite formatting differences. The algorithms used in fuzzy matching detect patterns and standardize entries by removing spaces, symbols, or extensions, enabling a clean, consistent dataset.
Understand, though, that phone numbers are not perfect unique identifiers – far from it. They can be incomplete, messy, and maybe obfuscated during data entry errors. If you’re using phone numbers as your unique identifiers, they must be standardized, deduped, and made complete.
Dates And The Challenge With Formats
The main problem with dates?
Formats.
Here are some common examples:
Imagine matching these formats!
Now, let’s add another layer to this.
Time.
For instance, if someone enters a birth date of April 1, 1990, from the West Coast of the United States at 4:45 p.m., the system may record it with a timestamp of 4:45 PM PST (or PDT, depending on the time of year). Now, when a user views that same date from a location across the international date line, the system could display the birth date as April 2, 1990.
Confusing? Absolutely!
Fuzzy matching systems help address this issue by standardizing date formats and resolving inconsistencies caused by time zones. Rather than relying on exact matches, fuzzy matching can be set to recognize dates as equivalent entries if they are within a 24-hour range or flagged for review if discrepancies arise. Additionally, it can identify patterns in time-stamped dates that frequently shift due to regional viewing, allowing the CRM to apply a standard date format (e.g., removing time zones entirely or converting to UTC) across all records. This approach creates a more accurate, unified view of date-related information, reducing confusion and errors in customer data.
Matching Location & Address Data
Address or location data has two challenges – dirty or noisy entries and having multiple identities tied to one location. For example, five members of a household will share the same address, and so will five thousand employees of an organization.
Because of this, address data is hardly used for fuzzy matching unless it is parsed or broken down to resolve specific errors. These are:
📌 Key Observations from Address Variations
✅ Street names should be standardized (e.g., “St.” vs. “Street”).
✅ City and state variations can cause mismatches (e.g., “LA” vs. “Los Angeles”).
✅ ZIP+4 formatting should be enforced for consistency in databases.
✅ Country names should use a single standard format (e.g., “USA” over “United States”).
✅ Latitude/Longitude data should be verified for precision in geolocation matching.
When matching address data, it’s always better to standardize entries and ensure there is as little discrepancy as possible. You can do this in WinPure at a component level, thereby preparing your data for address verification.
Address data can be verified against the official postal address code of a country. In the U.S. for example, addresses can be verified against the USPS (United States Postal Service) database, which ensures that addresses conform to standardized formats and are deliverable. This verification helps maintain consistency across records and improves data quality by correcting errors in address entries.
Email Addresses And Duplicate Records
Similar to phone numbers, email addresses are unique to individuals – however – an individual can have multiple unique email addresses. Imagine a customer having a personal email, a throwaway email, and a work email – all of which are registered within your CRM.
Fuzzy matching can help identify and consolidate multiple email addresses belonging to the same individual within a CRM. By using similarity algorithms, fuzzy matching can detect patterns across email domains or names associated with the same customer (e.g., matching “[email protected],” “[email protected],” and “[email protected]”). It can also account for slight variations or typos, such as “john.doe” versus “john_doe,” which might otherwise create duplicate records.
Once these similar entries are identified, fuzzy matching allows the CRM to link them to a single customer profile. This creates a unified view of the individual, enabling more accurate customer tracking and improving the quality of customer interactions by preventing redundant communication across multiple emails.
How To Fuzzy Match With Winpure: A Step-By-Step Guide
Fuzzy matching has traditionally relied on complex coding and algorithmic tuning using libraries like FuzzyWuzzy (Python), stringdist (R), and SOUNDEX (SQL). While these methods offer control over the matching process, they come with significant limitations. Extracting data, defining match parameters, fine-tuning similarity thresholds, and consolidating results often require weeks or even months of manual effort. Moreover, scripts lack scalability, requiring ongoing maintenance to handle evolving data structures, new CRM fields, or multilingual variations.
This is where WinPure transforms the process by eliminating coding complexities and offering a fast, scalable, and accurate fuzzy matching solution. With an intuitive interface, pre-built AI-powered algorithms, and seamless data integration, WinPure enables users to clean, deduplicate, and match records in minutes without needing programming expertise. Whether working with customer databases, CRM systems, or financial records, businesses can now eliminate duplicates, reduce false positives, and maintain data integrity effortlessly.
Now, let’s walk through how to perform fuzzy matching using WinPure.
✅ Choose the Files: Select the datasets you need to clean and match. This step involves identifying the data sources that require deduplication or integration. It could be customer lists, CRM databases, marketing lists, or any other data files that contain potentially redundant or inconsistent entries.
✅ Clean the Data: Standardize the format, correct typos, and ensure the data is up-to-date. Cleaning the data is crucial as it prepares the datasets for effective matching. This includes correcting spelling errors, standardizing address formats, and ensuring that all entries follow a consistent structure.
✅ Match Between Files: Perform matching within a single file or between two different files. This step identifies potential duplicates and inconsistencies within the selected datasets. For example, matching a customer list against a marketing database to identify overlapping records.
✅ Set Match Rules: Define criteria for matching, such as matching company names with address data to identify duplicates. Match rules help in specifying the attributes that need to be compared. For instance, matching based on both name and address can help ensure that different entries for the same customer are identified as duplicates.
✅ Tight Match (90-95%): Provides optimal results with fewer false positives. A tight match threshold is set to ensure high accuracy in identifying duplicates. For example, a 95% threshold means that only entries with very similar attributes (e.g., “John Smith” and “Johnathan Smith”) will be considered matches. This reduces the risk of false positives but may miss some potential matches.
Loose Match (80-85%): Increases the likelihood of false positives but may catch more potential matches. A loose match threshold allows for more variability in the matching criteria. For example, an 85% threshold might identify “J. Smith” and “Jon Smith” as matches. This approach can catch more possible duplicates but also increases the chances of false positives, where unrelated entries are incorrectly flagged as matches.
Tight Match Example: “Robert Brown” and “Bob Brown” might not be matched at a 95% threshold, but “Robert Brown” and “Robert B.” would be.
Loose Match Example: “Robert Brown” and “Rob Brown” might be matched at an 85% threshold, capturing more potential duplicates but with a higher risk of including false positives.
✅ Merge, Overwrite, Delete
Merge: Consolidate redundant information into one comprehensive record. For example, merging the addresses “456 Elm Street” and “456 Elm St.” into a single, standardized format.
✅ Overwrite: Make decisions between conflicting versions of data, such as updating Address B instead of Address A. For instance, if one record has an outdated address, the correct, updated address can overwrite the old one.
A fuzzy match solution like WinPure allows the user to create custom word libraries to avoid a false positive. Additionally, users can perform matches as many times as they want without corrupting the data.
See how easy that was?
Resolving a problem like this using manual methods or coding requires additional steps that do not guarantee accuracy. Moreover, it impacts efficiency. Your team is wasting time on redundant problems!
How To Minimize False Positives And False Negatives In Fuzzy Matching?
Fuzzy matching is only as good as its ability to balance precision (reducing false positives) and recall (reducing false negatives). False positives group unrelated records, corrupting data integrity, while false negatives fail to link true matches, leading to missed business insights. Optimizing both requires algorithm tuning, data preparation, and validation strategies—here’s how to do it effectively.
1️⃣ Standardize & Clean Data First
Dirty data causes both false positives and false negatives.
✅ Fix:
- Convert text to lowercase (“ROBERT” → “robert”).
- Remove punctuation & special characters (“J.K. Rowling” → “JK Rowling”).
- Standardize abbreviations (“Ltd” → “Limited”).
- Normalize accents & phonetics (“José” → “Jose”).
2️⃣ Use The Right Matching Algorithm
A one-size-fits-all approach fails. Choose the right algorithm based on data type.
Algorithm | Best For | Avoid When… |
Levenshtein | Typos & minor spelling errors | Large datasets (slow processing) |
Jaro-Winkler | Name variations (e.g., “Jon” ≈ “John”) | Generic text (over-matching risk) |
Soundex | Phonetic matches (e.g., “Catherine” ≈ “Katherine”) | Numeric or structured data |
Cosine Similarity | Product descriptions, documents | Short strings (names, codes) |
3️⃣ Set Smart Similarity Thresholds
A fixed threshold (e.g., 85%) doesn’t work for all data. Lowering it too much can lead to excessive false positives, while setting it too high may miss valid matches.
✅ Fix:
- Stricter (90-95%) for finance, legal, compliance (fewer false positives).
- Looser (80-85%) for marketing, CRM deduplication (fewer false negatives).
- Caution: Lowering the threshold to 80-85% can significantly increase false positives.
- Dynamic thresholding based on data characteristics helps fine-tune accuracy.
🔹 Example:
- At 95%, “3 Day Blinds” matches to “3 Day Blinds 4” and “3 Day Blinds 216” (similar but distinct).
- At 85%, “1st & Foremost Inc” matches to “1st UNI Meth Scout” and “1st Stop Food Market” (higher risk of false positives).
Lowering thresholds can help identify harder-to-catch matches (e.g., “4 Season Nails” vs. “4 Seasons Salon & Day Spa” at 89%) but requires additional validation.
4️⃣ Match Across Multiple Fields
Relying on a single field (e.g., name) is risky, as minor variations can lead to missed matches or incorrect groupings.
✅ Fix:
- Match using “Name” + “Email” + “Phone”, not just “Name”.
- Assign weights (e.g., “Email” = 70%, “Company” = 30%”).
5️⃣ Create Custom Word Dictionaries
Common terms & abbreviations skew results.
✅ Fix:
- Ignore generic terms (“Corp”, “LLC”, “Inc.”).
- Whitelist known valid matches (“Jonathan” ≈ “Jon”).
- Blacklist false positives (“No-Reply Emails” shouldn’t be matched).
6️⃣ Human Review For Edge Cases
Even the best algorithms make mistakes.
✅ Fix:
- Flag 80-90% similarity matches for review.
- Implement exception handling for high-risk merges.
- Use machine learning feedback loops to refine over time.
By cleaning data, choosing the right algorithms, adjusting thresholds, and adding manual review where needed, you can minimize errors and maximize accuracy in fuzzy matching.
Tight vs. Loose Match Examples
✅ Tight Match (95%):
- “Robert Brown” and “Bob Brown” might not be matched at 95%.
- “Robert Brown” and “Robert B.” would be matched.
✅ Loose Match (85%):
Lowering thresholds to 85% can capture more duplicates but increases false positives significantly.
Example of an 85% threshold:
Match % | Name |
---|---|
100.00% | John Verzi |
88.02% | John Gray |
86.04% | John Murphy |
85.30% | John Garrard |
88.20% | John Stromback |
86.53% | John Kirchgesler |
86.04% | John Tinson |
86.83% | John Bowden |
In this case, “John Verzi” should not be matched with “John Garrard” or “John Clardy,” but at 85%, they may be grouped together.
Use Cases Of How Businesses Solve Data Challenges With Fuzzy Matching
Organizations across industries struggle with duplicate records, inconsistent data formats, and fragmented datasets. Here are three real-world use cases where businesses applied fuzzy matching to overcome these challenges.
1️⃣ Drinxsjobeck: Managing Large, Messy Datasets Under Tight Deadlines
📍 Industry: Beverage Supply Chain
⇒ Challenge: Cleaning and deduplicating massive datasets with high error rates
The Problem:
DrinxSjobeck, a major beverage supplier, faced a massive data consolidation task within a strict deadline. The company had to merge two enormous datasets containing duplicate and inconsistent customer records. Manual cleanup methods—spreadsheets and SQL scripts—were too slow and error-prone. The data was riddled with misspelled names, inconsistent formatting, and outdated records, making it difficult to process orders accurately.
The Solution:
DrinxSjobeck adopted a fuzzy matching approach to:
✔ Identify duplicate customer records despite variations in spelling and formatting.
✔ Process data 70% faster, allowing the team to meet deadlines.
✔ Improve data visibility, enabling proactive data standardization for future operations.
Results:
- Data processing time reduced by 70%, allowing smooth operations.
- Significant reduction in manual effort, freeing up IT resources for strategic initiatives.
- More accurate customer records, leading to improved order fulfillment and fewer errors.
2️⃣ Global Fmcg Brand: Replacing A Legacy Tool For Better Data Matching
📍 Industry: Consumer Goods (FMCG)
⇒ Challenge: Finding a fast, accurate, and scalable data matching solution
The Problem:
A leading FMCG company needed to replace its outdated data matching software, which was no longer supported. The tool was crucial for supply chain management, ensuring clean and deduplicated product and supplier data. Without a replacement, data discrepancies would lead to inaccurate reports, supply chain inefficiencies, and increased operational risks.
The Solution:
The company implemented a more flexible fuzzy matching solution, which:
✔ Delivered high-accuracy match results within minutes instead of hours.
✔ Provided customizable matching rules, ensuring precision in data consolidation.
✔ Allowed seamless transition from the old system without workflow disruptions.
Results:
- Eliminated duplicate product and supplier records, improving inventory accuracy.
- Reduced data processing time, enabling faster decision-making.
- Improved operational efficiency, preventing costly errors in supply chain management.
3️⃣ German Agriculture Machinery Brand: Improving Data Quality For Smarter Decision-Making
📍 Industry: Agricultural Engineering
⇒ Challenge: Standardizing inconsistent data across multiple systems
The Problem:
A leading agriculture machinery manufacturer was dealing with data inconsistencies across multiple departments. Their databases contained duplicate entries, inconsistent product information, and missing fields, making it difficult to analyze equipment performance, track resources, and optimize production. Existing methods were slow and unreliable, often requiring manual intervention.
The Solution:
By implementing a fuzzy matching approach, the company:
✔ Standardized product and supplier data, ensuring accuracy in reporting.
✔ Eliminated duplicate records, preventing costly errors in procurement and inventory.
✔ Enabled data validation and enrichment, improving the reliability of business insights.
Results:
- More accurate inventory and supplier records, reducing operational delays.
- Improved equipment tracking and maintenance scheduling, boosting efficiency.
- Greater data consistency, leading to better resource allocation and sustainability practices.
Across industries, businesses that prioritize data quality gain a competitive edge by improving operations, reducing costs, and increasing revenue.
Comparative Analysis: Choosing The Right Data Matching Approach For Your Business
Whether it’s customer records, financial data, or supply chain information, choosing the right method can impact accuracy, efficiency, and business outcomes. Let’s compare the effectiveness and practical use cases of data matching approaches.
To Conclude: Fuzzy Data Match is the Backbone of Data Quality!
Fuzzy data matching is not a novel concept, however, it has gained popularity over the past few years as organizations are struggling with the limitations of traditional de-duplication methods. With an automated solution like WinPure, you can save hundreds of hours in manual effort and resolve duplicates at a much higher accuracy level without having to worry about building in-house code algorithms or hiring expensive fuzzy match experts.
Watch the video to see how WinPure’s fuzzy match tool can help solve critical duplication challenges.