Table of Contents
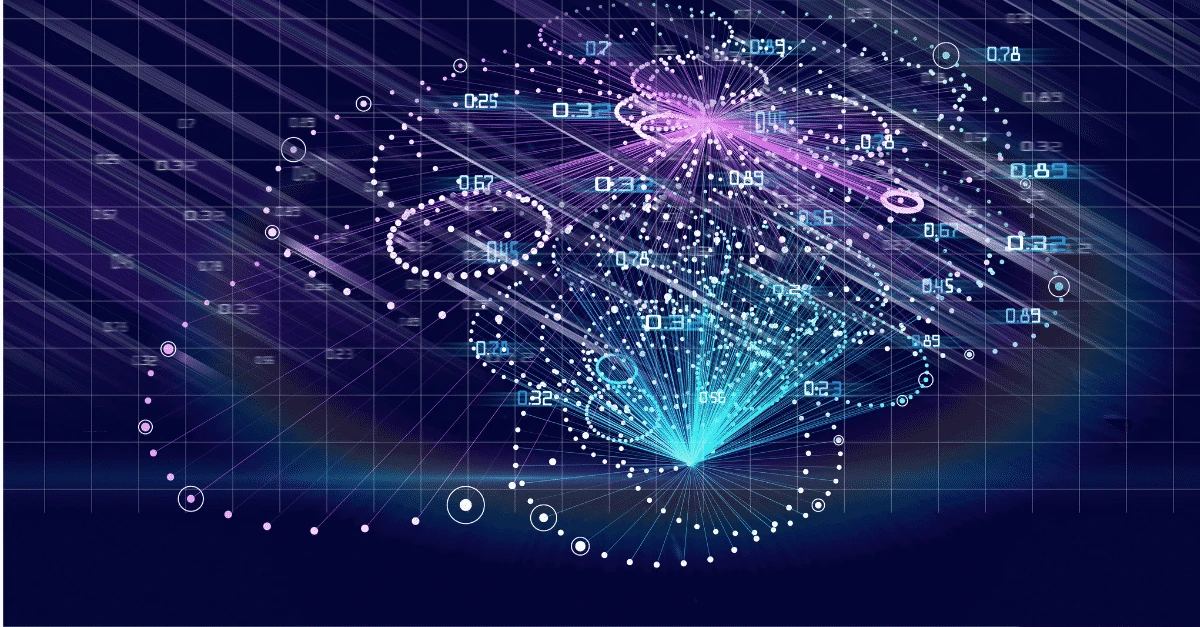
Do you know which data quality dimensions are most critical to your operations & how well your current data measures up?
Many businesses are sitting on mountains of data but still can’t trust it because they’re not tracking the right data quality metrics. As they race to implement AI & automation, many are hitting a wall with data migration & Master Data Management (MDM) projects. The reason? Poor data quality.
Learning how to track data quality metrics will help save money in your business and figuring out how to measure data quality does not need to be complicated. The best approach combines common data quality dimensions and data quality KPIs to calculate business results. From that point, you build trust that your business data will perform well in the right context at the right time.
Read further to learn more and to see how measuring data quality metrics works through four examples, showcasing real-world applications that highlight the importance of maintaining high-quality data across various dimensions.
What Are Data Quality Metrics?
Data quality metrics provide an objective way to measure whether your data is supporting or hindering your business operations. However, they go beyond surface-level assessments like completeness or accuracy. Realistically, data quality metrics are about understanding the cost of poor data on decisions, workflows, and long-term business strategy.
For example, accuracy might seem straightforward, but it’s not just about whether the data is right. It’s about whether that accuracy has a direct impact on outcomes. If a CRM contains 95% accurate customer details but those 5% inaccuracies exist among high-value clients, the damage is disproportionately large. Therefore, you can’t just look at the numbers—you need to dig into the implications of those inaccuracies.
Data quality metrics can also be used to evaluate data lineage. How has your data evolved through the different systems and transformations it’s been subjected to? What’s the impact of these transformations on the integrity of the data? These are harder questions that metrics help uncover. Without these measurements, businesses are often blind to data corruption or inconsistency as it flows through various processes.
Consider a pharmaceutical company that relies on clinical trial data to get approval for new medications. If timeliness is compromised at any point—perhaps data on patient reactions isn’t reported quickly enough—there could be delays in discovering side effects or even dangerous drug interactions. Now, imagine the ripple effect. The delay in identifying those risks could result in regulatory non-compliance, delayed approvals, and worse, putting patients at risk. Tracking metrics like timeliness and consistency at every stage of the trial ensures that data is available exactly when needed to make critical decisions that affect not just the business, but human lives.
In this context, data quality metrics offer visibility into unseen risks, uncover operational inefficiencies, and provide the foundation to make decisions you can trust.
How Do You Define Data Quality?
The precise definition of data quality refers to the overall state of qualitative or quantitative pieces of information or data based on measurable attributes like accuracy, completeness, consistency, timeliness, and validity. High-quality data is data that reliably supports your business processes and decision-making by delivering insights you can trust. Essentially, data quality determines whether the information you rely on is fit for its intended purpose.
To put it simply, data is considered high-quality if it consistently produces the correct outcomes for the same inputs and under similar conditions. If your data can’t be trusted to do this, it’s not serving its purpose.
For example, imagine a retail company that relies on customer data to drive personalized marketing campaigns. If that data is inaccurate—perhaps customers’ addresses are outdated or purchase histories are incomplete—the company risks sending promotional offers to the wrong individuals or missing opportunities to target key customer segments. As a result, not only is money wasted on ineffective marketing, but the customer experience is also impacted, leading to reduced customer loyalty.
Furthermore, high data quality means that you can be assured of the same results as previously demonstrated, given the same situation and inputs. Data quality tools such as WinPure are vital in achieving the level of data quality necessary for a profitable business that wishes to see continued growth.
Why Do Data Quality Metrics Matter?
Data quality metrics are the heartbeat of your business operations. When data is unreliable, every decision that follows is on shaky ground.
Take CRM systems, for example. Many businesses assume their CRM data is solid, but without regular checks on accuracy and completeness, they can quickly lose track of important customer information. A junior analyst might discover that key fields—like phone numbers or emails are missing or outdated. This might seem minor, but it’s like a leak in a dam: small at first, but it can lead to bigger problems like missed follow-ups or failed marketing campaigns.
For data or information managers, these metrics are not abstract concepts—they’re the difference between smooth operations and operational chaos. Metrics give you visibility into what’s right and what’s wrong, so you can make decisions that are grounded in truth, not assumptions.
Without measuring these aspects, you’re driving blind.
What Are the 6 Dimensions of Data Quality?
Organizations agree that data quality falls into six core dimensions or characteristics:
- Data Completeness: Completeness is about ensuring that the data collected is actually the right data for the task. Organizations often gather enormous volumes of data but neglect to measure the completeness of the essential data points that drive real insights. A lack of critical data, even in small portions, skews results and impacts the ability to derive actionable intelligence. What’s seldom discussed is how even incomplete metadata or contextual information can degrade decision-making processes.
- Data Accuracy: Accuracy is about ensuring data is precisely aligned with its intended purpose. The conversation around data accuracy often stops at correcting errors, but true accuracy involves aligning data with evolving business needs. What’s rarely mentioned is the cumulative impact of persistent inaccuracies such as systematic biases in data collection methods or assumptions built into data pipelines. This misalignment creates ongoing strategic miscalculations, where the damage is not immediately visible but reveals itself over time through misinformed decisions.
- Timeliness: Timeliness is often narrowly interpreted as data being up to date, but in reality, it’s about the relevance of the data within the specific operational window. Data that’s technically accurate but not timely loses value in dynamic environments. Few organizations talk about the misalignment between data acquisition cycles and the actual decision-making timelines, leading to bottlenecks in strategic processes. Timeliness is about understanding how the latency of data impacts real-time operations and the often-overlooked friction this creates across departments.
- Uniqueness: Uniqueness addresses more than duplicate records. It’s about ensuring singularity across systems and datasets. This isn’t just an issue of database hygiene but avoiding fundamental contradictions in critical operational data. Businesses need to think about how duplicate data can result in multiple “truths” within the same organization.
- Consistency: Consistency is less about matching data formats and more about ensuring that the data tells the same story across different platforms, systems, and departments. Most organizations fail to address that data that is technically consistent but misaligned with operational contexts. This is where the real breakdown happens: departments pulling data from different sources and arriving at contradictory conclusions. Seldom discussed is the risk of false consistency, where data appears uniform on the surface but reveals deeper discrepancies once it’s used across diverse functions.
- Validity: Validity is often reduced to data conforming to predefined formats or rules, but it’s really about whether the data is fit for purpose. The challenge that goes unnoticed is that many businesses rely on outdated or overly simplistic validation rules, which miss critical nuances of business models. Rigid validation processes can exclude valuable data that doesn’t fit the mold, while allowing incorrect but technically valid data through. This lack of flexibility in defining validity can result in costly rework, operational delays, and flawed compliance strategies.
Relate Reading: To learn more about data management, then head to our Master Data Management (MDM) Guide where we cover everything there is to know about the Master Data Management process, including MDM architecture and framework.
What are Examples of Quality Metrics?
Quality metrics are essential for resolving conflicts between different parts of an organization, like in the healthcare industry where IT and business teams often view data quality differently. Consider the example of a patient registration report run on the 15th of the month. It shows fewer patient registrations than a report rerun on the 30th. The business team might flag this as an issue of data completeness, suspecting that the initial report didn’t capture all the relevant data. They rely on this information to forecast patient loads, manage staff, and allocate resources effectively.
Meanwhile, the IT team might argue that the report is timely and accurate based on the data available on the 15th, and that any additional registrations that appear in the report on the 30th are simply due to the natural delay in processing. From their perspective, the report is functioning as intended, given the expected data input timeline.
Here’s where data quality metrics step in to clarify. Both sides may be right depending on what metric you’re using to evaluate the data. Completeness is about ensuring all patient data is captured by the end of the month, but timeliness refers to the report’s ability to provide actionable data when needed, even if some records arrive later.
This situation highlights the need to align on the relevant quality metrics—in this case, balancing timeliness for operational efficiency with completeness for long-term planning. By measuring both dimensions accurately, organizations can understand where data gaps exist and make informed decisions without internal conflicts.
DATA QUALITY KPIS
Here enters data quality KPIs or key performance indicators, necessary in understanding data quality goals. Data quality KPIs connect business objectives or KPIs to different data quality dimensions, such as accuracy.
In the example above, the business defines patient registrations data quality KPI as a complete patient list from day 1 to day 30. IT defines data quality as a comprehensive patient list from the day printed (patients registered on the 20th would not show up on a report run on the 15th).
As you can see, data quality measures require the same understanding of data quality KPIs according to data quality dimensions. Furthermore, in considering many data quality dimensions and business requirements, you will need several different kinds of data quality measurements to assess whether you have the data quality you need.
Data quality measures describe the entire set of values calculated from how well data quality KPI’s meet data quality dimensions. The closer the data quality scores to the desired results, where and when they are needed, then the more organizations can be confident in their data.
How Do You Measure Data Quality Metrics?
Measuring data quality metrics starts with identifying the key business needs and the data quality dimensions that impact them such as accuracy, completeness, timeliness, or consistency. It’s not enough to measure these metrics in isolation, they must be relevant to the specific business objectives and use cases.
The first step involves calculating objective functions. Things like counts, averages, sums, or percentages that correspond to the data quality KPIs you’ve established. For example, when assessing completeness, you might count how many required data fields are missing or unpopulated. But understanding context is crucial. What’s missing, why is it missing & how does it affect operations?
Take patient registrations in healthcare: if data completeness is assessed purely by counting missing fields, you’re missing the bigger picture. You need to analyze what specific fields (like check-in dates or lab results) are incomplete, why those gaps exist, and how that affects patient care, scheduling, and compliance.
Next, you must validate the data against business processes. Let’s say you’re tracking how many patients followed through on a recommended screening. The count of registered patients is a metric, but to fully understand its impact, you also need to assess why certain patients didn’t follow through. This gathering real-world feedback like conducting surveys or checking for cross-registration in other clinics.
Last but not least, ongoing monitoring is essential. Measuring data quality isn’t a one-time task. Continuous validation, adjustment, and feedback loops help keep data quality metrics aligned with business needs.
The Four Data Quality Metrics Examples
To get a better sense about applying data quality metrics to your data quality KPIs, we have used these examples below to illustrate how to measure data quality metrics.
Keep in mind these data quality metrics examples cover some but not all data quality measurements. These cases will guide you in setting up and using data quality metrics relevant to your business goals and the characteristics you wish to measure.
Here is the breakdown of data quality metric examples
Example 1
Dimensions: Accuracy, Timeliness
Data Quality KPIs: Fast and accurate identification of COVID-19 from those with concerning coughs
Data Quality Metrics: Number of people with a problematic cough that did not register in a hospital or clinic and had inaccurate data. The time gap cellphone users with a bad cough that took to enroll for a COVID-19 test.
How To Calculate: First combine all the data in the data systems and search for any required data cells not populated. Check the number of patient registrations that have positive COVID-19 test results at a particular clinic. Check this patient registration list in the tracker with a clinic. Note any discrepancies. Find the time gaps from a person notified about their cough to register at a clinic over one month.
Quality Metrics Example Case Study: WinPure Data Matching In Ground-Breaking COVID-19 Tracker Project
Example 2
Dimensions: Validity, Uniqueness
Data Quality KPI: A single view of unique, active Centura Health donors with no duplicated identifying data.
Data Quality Metrics: The count of duplicated identities should be 0. The number of identified Centura Health donors. Count of individuals falsely identified as a donor. Feedback from customers that they have received duplicate mail.
How To Calculate: Count the number of duplicate identities that show up in the view. It should be at 0. Total the number of records with cleansed data in an hour after automation vs. manual processes. Find the percentage of help desk tickets requiring resolution for duplicate mailings.
Quality Metrics Example Case Study: Fast & Accurate Data Matching Saves Precious Time
Example 3
Dimensions: Consistency, Uniqueness
Data Quality KPI: Over 162,000 clean, correct, and standardized address data flow to the Customer Relationship Management (CRM) database in a standard, accurate format.
Data Quality Metric: Percentage of address data changed from one system to the CRM. The number of duplicate records in the CRM.
How To Calculate: Count the number of addresses where the critical data changed from one system to another. Count duplicate mailings sent, as reported by customers and the system, and compare them to duplicate records in the CRM.
Quality Metrics Example Case Study: Address Verification Provided Significant Cost Savings
Example 4
Dimensions: Completeness, Accuracy
Data Quality KPI: Ticket sales data in Birmingham Hippodrome matches have the same data retrieved by other agencies that sell tickets.
Data Quality Metrics: The number of ticket records with complete data retrieved by other agents matches the same number in Birmingham Hippodrome’s database. The same ticket data contents from a data profiled in Birmingham Hippodrome’s database matched that found in the other agencies.
How To Calculate: Check the Birmingham Hippodrome’s database for any empty values required by both the internal IT and the ticket agencies. Of the duplicate mail sent to customers, check the percentage of duplicate records in the Birmingham Hippodrome’s database.
Quality Metrics Example Case Study: Top Performance for WinPure’s Clean and Match Tool.
Final Words On Data Quality Metrics
Research has found agreed-upon data metrics according to data quality dimensions: completeness, accuracy, timeliness, uniqueness, consistency, and validity. But these metrics need to pertain to your business context to earn business trust in its data and its correct operations over the right context at the right time. Connect your data quality metrics to data quality KPIs and the six data quality dimensions to better understand your data quality.